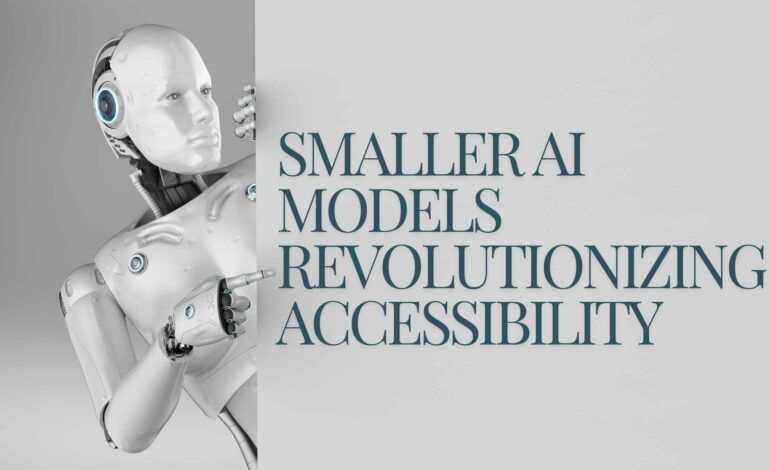
Smaller AI Models Revolutionizing Accessibility
Artificial Intelligence (AI) is transforming industries, and its widespread adoption is creating new opportunities and challenges. Traditionally, AI required significant computational resources, making it accessible primarily to large corporations with the financial and technological infrastructure to support it. However, the rise of smaller, more efficient AI models is democratizing AI, making it more accessible to startups, small businesses, and individual developers. One notable example is Mistral’s “Mixtral,” which is leading the charge in offering high-performance AI with reduced hardware requirements.
In this article, we will explore how smaller AI models are revolutionizing accessibility, enabling more users to harness AI’s power without the need for massive infrastructure. We’ll delve into the technical advancements driving this trend, the impact on various industries, and what the future holds for accessible AI.
Table of Contents
Introduction to AI Accessibility
Artificial Intelligence has long been seen as a technology reserved for tech giants and well-funded institutions. The complexity and cost of running AI models, especially large-scale ones, have traditionally been barriers to entry for smaller entities. However, a new wave of innovation is breaking down these barriers, making AI accessible to a wider audience.
The evolution of smaller AI models is one of the key factors driving this change. These models offer similar performance to their larger counterparts but with significantly lower hardware requirements. This shift is enabling more startups, developers, and individual researchers to integrate AI into their projects, fueling innovation across various fields.
The Rise of Smaller AI Models
Compact Models vs. Large-Scale Models
Historically, AI development focused on creating larger models with billions of parameters to achieve higher accuracy and performance. These models, while powerful, required vast computational resources, including high-end GPUs, large-scale cloud infrastructure, and significant energy consumption. For example, models like OpenAI’s GPT-3 and GPT-4 required massive server farms to train and run.
In contrast, smaller AI models are designed to deliver high performance with fewer parameters, reducing the need for extensive hardware. These compact models, such as Mistral’s “Mixtral,” can outperform much larger models on certain benchmarks while requiring a fraction of the resources. This is achieved through innovations in model architecture, optimization techniques, and efficient use of training data.
How Mistral’s “Mixtral” Model is Leading the Way
Mixtral’s Technical Advantages
Mistral’s “Mixtral” model is a prime example of how smaller AI models are changing the game. Mixtral is a mixture of experts (MoE) model that integrates eight neural networks, each with seven billion parameters. Despite its relatively small size, Mixtral outperforms models like Llama 2 with 70 billion parameters on most benchmarks. This is a significant achievement, as it demonstrates that smaller models can compete with, and even surpass, much larger models in terms of performance(IBM – United States).
One of Mixtral’s key technical advantages is its use of multiple smaller networks, allowing for more efficient processing and faster inference speeds. This not only reduces the computational burden but also makes the model more adaptable to various tasks, from natural language processing to computer vision.
Performance and Efficiency
Mixtral’s ability to match or exceed the performance of larger models while operating at six times the speed is a game-changer. For startups and individuals who cannot afford the infrastructure required to run massive models, Mixtral offers a viable alternative. Its efficiency means that it can run on more affordable hardware, such as standard GPUs or even edge devices.
This level of performance and efficiency is opening up AI to a broader range of users, from independent developers to small businesses looking to integrate AI into their operations without significant investment in infrastructure.
Democratizing AI for Startups and Individuals
Lowering Barriers to Entry
One of the most significant impacts of smaller AI models is the lowering of barriers to entry for AI development. Startups, small businesses, and individual developers often lack the resources to invest in the high-end hardware and cloud infrastructure required by traditional large-scale models. Smaller models like Mixtral, which can run on more modest hardware, make AI development accessible to these groups(Digital Adoption).
For example, a small business could deploy an AI-powered customer service chatbot or a recommendation engine using a compact model without needing to rent expensive cloud servers. This democratization of AI is enabling more businesses to harness AI’s power to drive innovation, improve efficiency, and compete in the marketplace.
Enabling Innovation for Small Businesses
By making AI more accessible, smaller models are also fostering innovation among small businesses. These businesses can now leverage AI to solve problems, automate tasks, and create new products and services that were previously out of reach. For instance, a healthcare startup could use a smaller AI model to analyze medical data, or an agricultural business could use AI to optimize crop yields through predictive analytics(IBM – United States).
This trend is leveling the playing field, allowing smaller players to compete with larger companies that have traditionally dominated the AI space.
Applications of Smaller AI Models
Edge Computing and IoT
Smaller AI models are particularly well-suited for edge computing and Internet of Things (IoT) applications. These models can run on devices with limited computational power, such as smartphones, sensors, and other IoT devices. This enables real-time data processing and decision-making at the edge of the network, reducing the need for constant communication with centralized servers(IBM – United States).
For example, a smart home system could use a compact AI model to process voice commands locally, improving response times and reducing dependence on cloud services. Similarly, IoT devices in industrial settings could use AI to monitor equipment and predict maintenance needs without the need for constant data transmission to the cloud.
Healthcare, Agriculture, and Finance
Beyond edge computing, smaller AI models are finding applications in various industries, including healthcare, agriculture, and finance. In healthcare, AI models can assist in diagnosing diseases, analyzing medical images, and personalizing treatment plans. The reduced hardware requirements of smaller models make them more accessible to clinics and hospitals with limited resources(Digital Adoption).
In agriculture, AI can help optimize planting schedules, monitor crop health, and predict yields, all while running on affordable hardware in rural areas. In finance, AI models can be used for fraud detection, risk assessment, and customer service, providing smaller financial institutions with the tools to compete with larger banks.
Challenges and Limitations
While smaller AI models offer numerous advantages, they are not without challenges. One limitation is that while they can perform well on specific tasks, they may not match the versatility of larger models across a wide range of applications. Additionally, developing and fine-tuning these models still requires expertise in AI and machine learning, which can be a barrier for some users.
There is also the issue of data privacy and security. While smaller models can be run locally, reducing the risk of data breaches, they still require careful handling of sensitive data, particularly in industries like healthcare and finance.
The Future of AI Accessibility
The trend toward smaller AI models is likely to continue as more developers and businesses recognize the benefits of accessible AI. Innovations in model architecture, optimization techniques, and hardware will further drive this trend, making AI even more accessible to a broader audience.
In the future, we can expect to see even more sophisticated AI applications running on smaller devices, from personal assistants to autonomous vehicles. As AI becomes more ingrained in everyday life, the ability to deploy powerful models on affordable hardware will be crucial for ensuring that the benefits of AI are shared widely.
Frequently Asked Questions (FAQs)
Q1: What makes smaller AI models more accessible?
Smaller AI models are designed to require fewer computational resources, making them accessible to users who do not have access to high-end hardware or cloud infrastructure. This allows startups, small businesses, and individual developers to integrate AI into their projects without significant investment.
Q2: How do smaller AI models compare to larger models in terms of performance?
Smaller AI models, like Mistral’s Mixtral, can match or even surpass larger models in terms of performance on specific tasks, all while operating at a fraction of the cost and speed. However, they may not be as versatile across a wide range of applications.
Q3: What industries are benefiting from smaller AI models?
Industries such as healthcare, agriculture, finance, and IoT are benefiting from smaller AI models. These models allow for real-time processing, automation, and data analysis, enabling businesses in these industries to leverage AI without needing extensive infrastructure.
Q4: What are the challenges of using smaller AI models?
Challenges include the need for expertise in AI and machine learning to develop and fine-tune models, as well as ensuring data privacy and security. Additionally, smaller models may not be as versatile as larger models across different applications.
Q5: What is the future of smaller AI models?
The future of smaller AI models looks promising, with continued innovations in model architecture and optimization techniques. These models are expected to become even more accessible, enabling a wider range of applications on affordable hardware.
Conclusion
Smaller AI models are revolutionizing accessibility by making AI more affordable and accessible to a broader audience. As these models continue to evolve, they will play a crucial role in driving innovation and democratizing the benefits of AI.